
Did you know there is a tremendous evolution upcoming for human resource practitioners? The integration of machine learning in recruitment has brought about a paradigm shift in hiring and talent management processes. This blog explores the multifaceted impact of machine learning, unveiling its role in reducing costs, enhancing efficiency, and fostering inclusivity throughout the employee journey.
From candidate screening to post-hire personalized training support, we delve into the statistics, applications, and future projections that illuminate the transformative power of machine learning in shaping the future of talent acquisition.
Impact of Machine Learning in Recruitment and Talent Management Processes Today!
The main challenges in recruitment and talent management have always revolved around finding and retaining the best talent assessments tool for the organization. Traditional methods of hiring often rely on human intuition, manual screening, and static metrics, which can be time-consuming, biased, and inefficient. Machine learning can help overcome these limitations by automating tedious tasks, analyzing large datasets, predicting outcomes, and providing insights that human recruiters might miss.
Some of the benefits of machine learning in recruitment and talent management are:

- Proactive talent sourcing: Machine learning can help identify potential candidates before they actively enter the job market, by analyzing their online profiles, social media activity, and career interests. This can help recruiters reach out to passive candidates and build a talent pipeline.
- Real-time market insights: Machine learning can provide real-time insights into the job market, such as talent shortages, salary trends, and future demand for specific roles. This can help recruiters adjust their strategies and offer competitive compensation and benefits to attract and retain talent.
- Personalized candidate journey: Machine learning can tailor the recruitment process to each candidate, by delivering personalized job ads, screening questions, and interview feedback. This can enhance the candidate experience and increase the chances of hiring the right fit.
- Retention predictions: Machine learning can analyze employee data and behavior to predict which hires are likely to stay and which might be considering a move. This can help recruiters and managers intervene early and prevent turnover.
- Skill gap analysis: Machine learning can identify the skills of current employees and compare them to market trends and organizational needs. This can help recruiters and managers focus on targeted training and development, and plan for succession and career growth.
Machine Learning assists employers in gaining a deeper understanding of candidates and employees. This understanding enables them to develop suitable onboarding, development, and training programs especially those focused on enhancing attention to detail in employees. Machine learning has remarkably improved the accuracy of hiring and managing talent across the globe.
Machine Learning in Employee Journey:
Machine Learning revolutionizes the employee journey, shaping pre-hire by automating recruitment tasks and predicting candidate success. In the post-hire phase, it aids in talent retention with predictive analytics for attrition risks and personalized learning plans, optimizing HR processes and talent management.
Pre-Hire
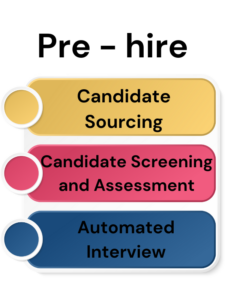
- Candidate Sourcing
The majority of employers, about 56%, use online platforms and machine learning to find candidates. They do this by analyzing patterns and improving their methods of discovering potential employees.
Furthermore, 46% of recruiters embrace the capabilities of artificial intelligence, particularly machine learning models, to craft compelling and targeted job descriptions. These models enhance the precision of language, ensuring job descriptions resonate effectively with the desired talent pool, ultimately optimizing the recruitment process.
Organizations are increasingly adopting sophisticated algorithms and machine learning models to streamline candidate sourcing, screening, and selection. These technologies enhance efficiency by automating repetitive tasks, enabling recruiters to focus on strategic aspects of talent management.
- Candidate Screening and Assessment
63% of decision-makers prioritize data-driven strategies when hiring new candidates. Why? So They can use data and evidence to assess candidates, emphasizing the importance of numbers and analyzing fitment without any human errors. This trend reflects mindful hiring that is assisted by data-driven decision-making in hiring.
- Automated Interview
37% of interviewers are using platforms that offer automated interview services, especially during the first interaction with candidates. Now, let's look into the role of ML in different aspects of automated interviews:
- Analyzing Video Interviews: ML models can analyze facial expressions, voice tone, and word choice in video interviews accurately. These models can identify skills and personality traits with 70% accuracy.
Natural Language Processing (NLP): NLP technology helps in understanding and assessing the linguistic nuances of candidates' responses. It evaluates communication skills, clarity, and the relevance of the information provided.
Post-Hire
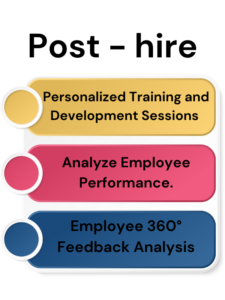
- Personalized Training and Development Sessions
82% of organizations use learning management systems (LMS) for personalized employee training and workforce development.
This widespread adoption reflects a strategic shift toward more efficient and tailored learning experiences. Learning management systems help organizations customize content, track progress, and adapt training for a skilled workforce.
- Analyze Employee Performance.
81% of HR professionals are thinking about changing the way they evaluate employees' performance in the workplace.
This shift suggests a growing recognition of the limitations inherent in conventional approaches. Organizations are exploring alternative methods that prioritize continuous feedback, real-time assessments, and a more dynamic understanding of employee contributions.
HR professionals aim to create adaptable systems that meet the current needs of employees and employers.
- Employee 360° Feedback Analysis
The global 360-degree feedback software experienced a remarkable average growth rate of 9.8% in 2020. This surge shows a significant increase in the use of feedback systems that evaluate employee performance from different angles.
Companies worldwide recognize the significance of obtaining feedback from various individuals. These individuals include colleagues, bosses, and team members. The purpose is to gain a better understanding of an employee's strengths and areas for improvement.
This growth underscores a shift towards more sophisticated and inclusive feedback mechanisms in talent management strategies.
How are Hiring Managers Already Using Machine Learning in Recruitment?
Employers, encompassing 58%, believe machine learning enhances their decision-making prowess in hiring. Currently, 73% of recruiters are integrating AI into their hiring processes. Furthermore, 69% acknowledge its positive impact on the overall hiring experience.
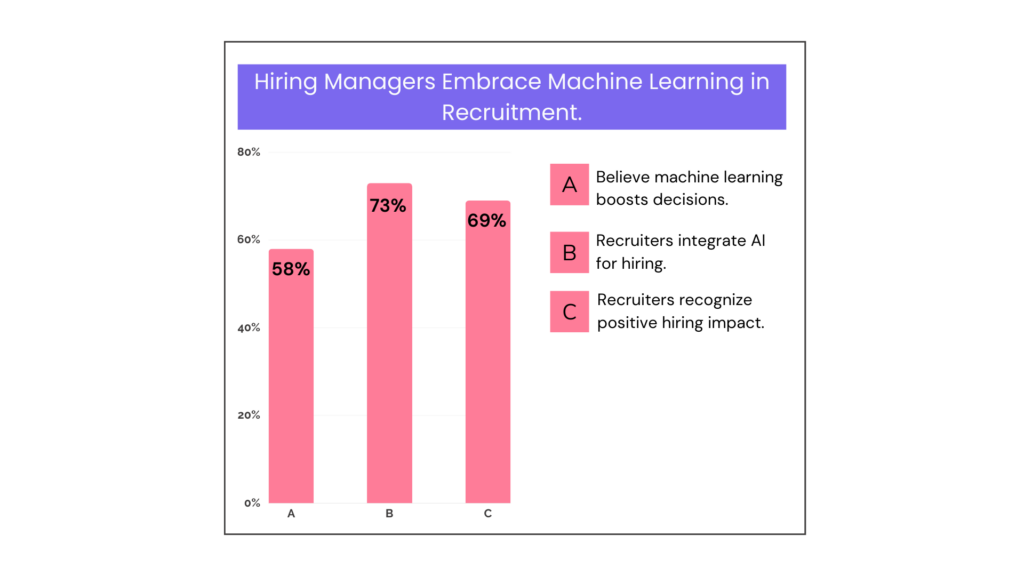
Hiring managers utilize Machine Learning in several different areas, including:

1. Job Advertising
Research suggests that machine learning algorithms play a significant role in improving the effectiveness of job advertising. 87% of recruiters expect to use machine learning in future recruiting, and 64% already use it in their processes.

Machine learning plays a pivotal role in optimizing various aspects of job advertising. By analyzing historical data, algorithms can target job advertisements more effectively, ensuring they reach candidates whose profiles align closely with the desired qualifications. Additionally, machine learning enables the personalization of job recommendations based on individual candidate behaviors and preferences.
The technology helps place ads on the best channels, improve the talent acquisition process with analytics, and automate resume screening. This technology helps in changing ads according to user data. It also supports diversity and inclusion efforts by identifying and correcting biases. Additionally, it enables ongoing monitoring and improvement of performance.
Through these applications, machine learning enhances the efficiency, precision, and inclusivity of the job advertising process, ultimately leading to improved hiring outcomes.
Machine learning is important in changing job advertising, making it more efficient, and improving recruitment outcomes.

2. Resume Screening
Machine learning-based resume screening improves candidate shortlisting accuracy by 25%, according to research from the Journal of Applied Psychology.
This revolutionary approach to talent acquisition offers substantial benefits, such as:
- ML systems save time by quickly reviewing resumes, reducing recruiters' workload by up to 80% compared to manual methods.
- Implementing ML can lead to cost savings of 10-15% in the recruitment process.
- ML-based systems appear with up to 90% accuracy in finding good candidates, beating the 70% achieved by humans.
- ML algorithms mitigate implicit biases by objectively analyzing skills and experience based on predefined criteria, overcoming subjective human interpretation.
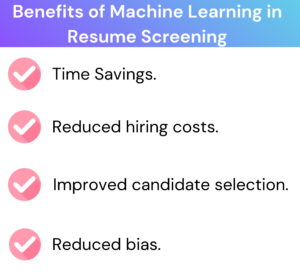
3. Candidate Assessment
Research indicates that incorporating machine learning into candidate assessments can significantly enhance the evaluation process. To achieve this, we consider both technical skills and soft skills. This holistic approach significantly enhances the accuracy of predicting candidate success.
The recruitment landscape is undergoing a profound shift with machine learning technology taking center stage in candidate assessment. A study from Oxford University discovered that machine learning models can accurately predict job success with 85% accuracy. Achieve this by prioritizing objective skills over subjective evaluations McKinsey's 2022 report highlighted ML's ability to find talented individuals by analyzing their skills and past performance data.
Leveraging Machine Learning for Enhanced Candidate Assessment:
- Application of ML: ML-driven assessments utilize diverse exercise data to create models predicting skill levels.
- Functionality: Interactive exercises generate performance data, analyzed by ML models for precise skill evaluation.
- Benefits: ML-guided adaptive testing ensures accurate assessments of candidates' abilities, reflecting their skill set.
- Analyzing the Video Interview:
- ML application: We train algorithms on video and audio data to recognize facial expressions, tone, and language patterns.
- Functionality: ML analyzes non-verbal cues, sentiment, and communication style during video interviews to assess soft skills.
- Benefits: ML-powered analyzing enhances objectivity in evaluating candidates, providing valuable insights beyond traditional assessments.
- Predictive Analytics:
- ML applications: It involves training models on historical data to actively identify factors contributing to candidate success.
- Functionality: ML algorithms predict a candidate's likelihood of success based on patterns found in past successful hires.
- Benefits: Predictive analytics streamline decision-making, guiding recruiters toward candidates more likely to excel in a given role.
- Cultural Fit Assessment:
- Application of ML: Algorithms analyze language patterns in company communications and employee interactions.
- Functionality: ML identifies linguistic cues indicating alignment with company values and culture.
- Benefits: Efficient cultural fit assessment ensures a candidate's compatibility with the organization's ethos and work environment.
By using machine learning in these online assessments, things get done faster, and organizations get powerful tools to make smart decisions. It helps them be more flexible and forward-thinking when it comes to finding and hiring the right people.
4. Candidate Engagement
Research emphasizes that organizations recognize the positive impact of personalized candidate engagement facilitated by machine learning, attributing it to an overall enhancement in the candidate experience.
70% increase in candidate engagement: Personalized communication and targeted content based on a candidate's interests and skills can significantly improve their overall experience.
In a 2023 Indeed study, machine learning automates and personalizes the candidate experience, handling tasks like emails, interview scheduling, and feedback. This tailored communication fosters a more valued and engaged experience for candidates throughout the hiring process.
- Workforce Planning
Longitudinal studies reveal that organizations embracing machine learning (ML) in their workforce planning witness substantial benefits, including reduced talent acquisition costs and enhanced long-term employee retention. The versatility of ML is evident in specific applications such as predicting labor demand, addressing skills gaps, optimizing scheduling, and tackling employee engagement challenges.
Applications of Machine Learning in Workforce Planning:
- Environmental Scan:
- ML Application: Analyze external data sources using ML algorithms to identify trends, industry shifts, and talent market dynamics.
- Benefits: Enhances the accuracy and speed of environmental scanning, providing real-time insights for informed decision-making.
- Strategic Planning:
- ML Application: Predictive analytics models analyze historical data to identify patterns, aligning workforce planning with strategic goals.
- Benefits: Assists in making data-driven decisions, ensuring alignment with organizational objectives.
- Future Requirements:
- ML Application: Predictive modeling forecasts future skill needs based on evolving business requirements and industry trends.
- Benefits: Provides proactive insights for anticipating and addressing future talent needs.
- Recruitment and Development:
- ML Application: Automated candidate screening using ML algorithms identifies the most suitable candidates based on historical hiring data.
- Benefits: Speeds up recruitment, improves candidate matching, and enhances the quality of hires.
Some More Findings That Reinforce ML's Impact on Employee Life Cycle:
Statistics emphasize the growing importance of ML in workforce planning, with 40% of companies already leveraging this technology.
Remarkably, companies adopting ML in workforce planning experience a commendable 10% reduction in labor costs, coupled with a 20% improvement in employee engagement and productivity. Moreover, ML contributes to a 50% reduction in the time required for critical workforce planning decisions.
Machine Learning's Pivotal Role in Talent Acquisition: Current Insights and Future Projections
In conjunction with these insights, 72% of recruiters anticipate the pivotal role of machine learning in talent acquisition over the next 3-5 years, and 62% of organizations have already integrated it into their recruitment processes. Notably, machine learning slashes time-to-hire by 50%, elevates candidate quality by 20%, and mitigates bias by 30%.
Anticipating its indispensability, 80% of HR leaders expect machine learning to be integral within the next five years, with a surge to 70% by 2025. As technology progresses, innovative applications in this pivotal domain are on the horizon.
Significant Applications of Machine Learning in Talent Acquisition:
- Chatbots for Candidate Support:
Implementation: Integrate chatbots into the recruitment process.
Functionality: Chatbots provide instant responses, offer job information and guide candidates.
Benefits: Enhances candidate experience with real-time support, ensuring informed and smooth navigation.
- Machine Learning Algorithms for Candidate Screening:
Implementation: Develop ML models based on historical hiring data.
Functionality: Analyze resumes and past performance to identify successful hire patterns.
Benefits: Improves efficiency by automating screening, allowing recruiters to focus on high-potential candidates.
- Personalized Outreach Strategies:
Implementation: Use ML to analyze candidate preferences.
Functionality: Tailor communication with personalized emails, job recommendations, and targeted content.
Benefits: Boosts engagement and builds a positive employer brand through customized interactions.
- Predictive Analytics for Dropout Prevention:
Implementation: Implement predictive analytics models using ML.
Functionality: Analyze candidate behavior to predict potential dropouts.
Benefits: Allows proactive intervention, reducing dropout rates and enhancing overall conversion.
These applications leverage machine learning to streamline recruitment, improve efficiency, and create a personalized and engaging journey for candidates, highlighting its current and growing significance in talent acquisition.
Conclusion:
In summary, the infusion of machine learning is reshaping HR practices, from refining job advertising to revolutionizing workforce planning. The statistics outlined underscore a pivotal shift toward agile, data-driven, and inclusive approaches in talent management.
As we traverse this evolving landscape, the growing impact of machine learning promises further innovation and efficiency in HR processes. The ongoing synergy between technology and human expertise is poised to redefine the future of talent acquisition and management.
To know more about how you can use the right psychometric assessments for your organization, call PMaps at +91 8591320212, email us at assessment@pmaps.in, or get in touch through our website at www.pmapstest.com.
